Hoping It's Not Too Late
Don't miss early signs of cancer and abnormalities with MEDAI Solutions
Tumor Diagnosis
Segmentation technology that distinguishes tumors from organs
CT Restoration
Support for surgery through complete CT restoration
Cancer Subtype Classification
Subtype classification technology that solves the problem of inaccurate tumor type classification
3D Visualization
Accurate 3D modeling technology that solves problems in conventional CT-based surgery
Post-Surgery Prognosis Prediction
Prognosis prediction model after surgery based on the generated 3D model
Research Achievements
Media
MEDAI Selected for Final Round of Deep Tech TIPS Program
MEDAI has been selected for the final round of the Ministry of SMEs and Startups' Deep Tech TIPS (Tech Incubator Program for startup Korea) program. The TIPS program is a private investment-led technology startup support initiative, where designated investment firms act as operators to provide investment, incubation, mentoring, and matched R&D funds from the government. The 'Deep Tech TIPS' program targets companies in the top 10 critical technology sectors for startups designated by the government, offering up to 1.7 billion KRW over three years (1.5 billion KRW for R&D and 200 million KRW for startup and marketing). MEDAI was selected in August 2024 for the bio sector of the Deep Tech TIPS program, gaining recognition for its innovative technology development in the field of medical AI. With this selection, MEDAI plans to accelerate its business expansion for R&D and global market entry.
08. 22. 2024Publication
Publication in Computers in Biology and Medicine (CIBM)
Abstract Multi-phase computed tomography (CT) has been widely used for the preoperative diagnosis of kidney cancer due to its non-invasive nature and ability to characterize renal lesions. However, since enhancement patterns of renal lesions across CT phases are different even for the same lesion type, the visual assessment by radiologists suffers from inter-observer variability in clinical practice. Although deep learning-based approaches have been recently explored for differential diagnosis of kidney cancer, they do not explicitly model the relationships between CT phases in the network design, limiting the diagnostic performance. In this paper, we propose a novel lesion-aware cross-phase attention network (LACPANet) that can effectively capture temporal dependencies of renal lesions across CT phases to accurately classify the lesions into five major pathological subtypes from time-series multi-phase CT images. We introduce a 3D inter-phase lesion-aware attention mechanism to learn effective 3D lesion features that are used to estimate attention weights describing the inter-phase relations of the enhancement patterns. We also present a multi-scale attention scheme to capture and aggregate temporal patterns of lesion features at different spatial scales for further improvement. Extensive experiments on multi-phase CT scans of kidney cancer patients from the collected dataset demonstrate that our LACPANet outperforms state-of-the-art approaches in diagnostic accuracy. <https://www.ncbi.nlm.nih.gov/pmc/articles/PMC10641799/>
06. 15. 2024Award
2nd Place in MICCAI AI Competition, a Prestigious Medical Society Worldwide
The Seoul St. Mary's Hospital-MedAI team announced on the 15th that they achieved excellent results at the world medical AI competition 'MICCAI 2023' hosted by the Medical Image Computing and Computer Assisted Intervention conference (MICCAI) recently. A joint team composed of a total of 6 researchers from Seoul St. Mary's Hospital and MedAI won second place after the AI company NVIDIA in the 2023 Kidney and Kidney Tumor Segmentation challenge (KiTS23), using a deep learning model. At this year's competition held in Vancouver, Canada, world-renowned companies and university research teams, including NVIDIA, competed in the field of AI algorithms that automatically segment the kidney area, kidney cancer area, and kidney cysts from abdominal CTs. Professor Hong Seong-hu of the Department of Urology at Seoul St. Mary's Hospital said, 'This MICCAI award proves that the Seoul St. Mary's Hospital-MedAI team's AI technology for diagnosing kidney cancer is of the highest global competitiveness and we will work harder to apply AI technology in the actual medical field.' <https://www.medipana.com/medician/view.php?news_idx=320520>
10. 08. 2023Certification
Certification of Compliance by Accredited Testing Organization KOIST
MEDAI has conducted a performance evaluation of the our AI model by the external certified testing organization KOIST, and we have received a pass judgment for all assessments.
09. 07. 2023Publication
Publication and Patent Acquisition in IEEE, a Top Authority Scientific Journal Worldwide
Multi-phase computed tomography (CT) is widely adopted for the diagnosis of kidney cancer due to the complementary information among phases. However, the complete set of multi-phase CT is often not available in practical clinical applications. In recent years, there have been some studies to generate the missing modality image from the available data. Nevertheless, the generated images are not guaranteed to be effective for the diagnosis task. In this paper, we propose a unified framework for kidney cancer diagnosis with incomplete multi-phase CT, which simultaneously recovers missing CT images and classifies cancer subtypes using the completed set of images. The advantage of our framework is that it encourages a synthesis model to explicitly learn to generate missing CT phases that are helpful for classifying cancer subtypes. We further incorporate lesion segmentation network into our framework to exploit lesion-level features for effective cancer classification in the whole CT volumes. The proposed framework is based on fully 3D convolutional neural networks to jointly optimize both synthesis and classification of 3D CT volumes. Extensive experiments on both in-house and external datasets demonstrate the effectiveness of our framework for the diagnosis with incomplete data compared with state-of-the-art baselines. In particular, cancer subtype classification using the completed CT data by our method achieves higher performance than the classification using the given incomplete data. <https://pubmed.ncbi.nlm.nih.gov/36327174/>
12. 08. 2022Publication
Publication in Nature Oncology, a Top World Journal in the Medical Field
In 2020, it is estimated that 73,750 kidney cancer cases were diagnosed, and 14,830 people died from cancer in the United States. Preoperative multi-phase abdominal computed tomography (CT) is often used for detecting lesions and classifying histologic subtypes of renal tumor to avoid unnecessary biopsy or surgery. However, there exists inter-observer variability due to subtle differences in the imaging features of tumor subtypes, which makes decisions on treatment challenging. While deep learning has been recently applied to the automated diagnosis of renal tumor, classification of a wide range of subtype classes has not been sufficiently studied yet. In this paper, we propose an end-to-end deep learning model for the differential diagnosis of five major histologic subtypes of renal tumors including both benign and malignant tumors on multi-phase CT. Our model is a unified framework to simultaneously identify lesions and classify subtypes for the diagnosis without manual intervention. We trained and tested the model using CT data from 308 patients who underwent nephrectomy for renal tumors. The model achieved an area under the curve (AUC) of 0.889, and outperformed radiologists for most subtypes. We further validated the model on an independent dataset of 184 patients from The Cancer Imaging Archive (TCIA). The AUC for this dataset was 0.855, and the model performed comparably to the radiologists. These results indicate that our model can achieve similar or better diagnostic performance than radiologists in differentiating a wide range of renal tumors on multi-phase CT. <https://www.nature.com/articles/s41698-021-00195-y>
06. 18. 2021Research and Business Partners
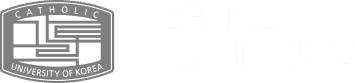

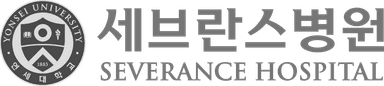
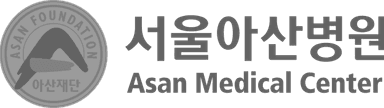

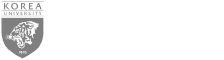
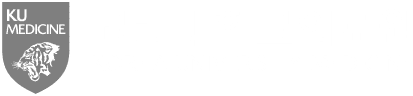
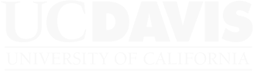

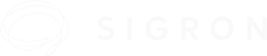
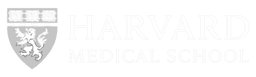