더 이상 너무 늦지 않기를 바랍니다
MEDAI의 솔루션을 통해 간과할 수 있는 초기 암과 이상징후들을 놓치지 마세요
종양 진단
종양과 장기를 구분해내는 Segmentation 기술
CT 복원
완전 CT 복원을 통한 수술 지원
암 아형 분류
부정확한 종양 유형 분류 문제를 해결하는 아형 분류 기술
3D 시각화
기존 CT 기반 수술에서의 문제점을 해결하는 정확한 3D 모델링 기술
수술 후 예후 예측
생성된 3D 모델 기반 수술 후 예후 예측 모델
연구성과
Media
MEDAI, 딥테크 팁스 프로그램 최종 선정
MEDAI가 중소벤처기업부의 딥테크 팁스(Deep Tech TIPS) 프로그램에 최종 선정되었습니다. TIPS(Tech Incubator Program for startup Korea) 프로그램은 민간투자주도형 기술창업지원사업으로, 운영사로 지정된 투자사 등을 통해 투자, 보육, 멘토링과 함께 R&D 자금을 정부에서 매칭하여 지원하는 사업입니다. '딥테크 팁스' 프로그램은 정부의 스타트업 10대 초격차 분야에 해당하는 기업을 대상으로 3년간 최대 17억 원(R&D 15억 원, 창업 및 마케팅 2억 원)을 지원하는 프로그램입니다. MEDAI는 2024년 8월 딥테크 팁스 바이오분야에 최종 선정되었으며, 의료 인공지능 분야에서의 혁신적인 기술 개발로 주목받고 있습니다. 이번 딥테크 팁스 선정으로 MEDAI는 향후 R&D 및 글로벌 시장 진출을 위한 사업 확장에 박차를 가할 예정입니다.
2024. 08. 22Publication
Computers in Biology and Medicine (CIBM) 논문 게재
Abstract Multi-phase computed tomography (CT) has been widely used for the preoperative diagnosis of kidney cancer due to its non-invasive nature and ability to characterize renal lesions. However, since enhancement patterns of renal lesions across CT phases are different even for the same lesion type, the visual assessment by radiologists suffers from inter-observer variability in clinical practice. Although deep learning-based approaches have been recently explored for differential diagnosis of kidney cancer, they do not explicitly model the relationships between CT phases in the network design, limiting the diagnostic performance. In this paper, we propose a novel lesion-aware cross-phase attention network (LACPANet) that can effectively capture temporal dependencies of renal lesions across CT phases to accurately classify the lesions into five major pathological subtypes from time-series multi-phase CT images. We introduce a 3D inter-phase lesion-aware attention mechanism to learn effective 3D lesion features that are used to estimate attention weights describing the inter-phase relations of the enhancement patterns. We also present a multi-scale attention scheme to capture and aggregate temporal patterns of lesion features at different spatial scales for further improvement. Extensive experiments on multi-phase CT scans of kidney cancer patients from the collected dataset demonstrate that our LACPANet outperforms state-of-the-art approaches in diagnostic accuracy. <https://www.ncbi.nlm.nih.gov/pmc/articles/PMC10641799/>
2024. 06. 15Award
세계 최고 권위 의료 학회 MICCAI AI 경진대회 2위 수상
서울성모병원-메드에이아이 팀은 인공지능 학술대회 의료영상 컴퓨팅 및 인터벤션 학술대회(MICCAI, The Medical Image Computing and Computer Assisted Intervention)에서 주최한 세계 의료 인공지능 경진대회 "MICCAI 2023"에서 최근 우수한 성적을 거뒀다고 15일 밝혔다. 서울성모병원과 메드에이아이 연구진 총 6명으로 구성된 연합팀은 딥러닝 모델을 활용해 신장과 신장암 분할 경진대회(KiTS23, The 2023 Kidney and Kidney Tumor Segmentation challenge)에서 인공지능 기업 NVIDIA에 이어 2위를 수상했다. 캐나다 밴쿠버에서 진행한 올해 대회에는 NVIDIA를 비롯해 세계 유수 기업 및 대학 연구팀이 참가해 복부 CT에서 신장 영역과 신장암 영역, 신장 낭종을 자동으로 분할하는 인공지능 알고리즘 분야에서 성능을 겨뤘다. 서울성모병원 비뇨의학과 홍성후 교수는 "이번 MICAAI 수상은 서울성모병원-메드에이아이 팀의 인공지능 신장암 진단 기술이 세계 최고 수준의 경쟁력을 갖추고 있음을 입증한 것으로 인공지능 기술이 실제 의료영역에 적용될 수 있도록 더욱 노력하겠다"고 말했다. <https://www.medipana.com/medician/view.php?news_idx=320520>
2023. 10. 08Certification
공인 시험기관 KOIST 시험 적합 판정
MEDAI는 외부 공인 시험기관 KOIST에서 인공지능 모델에 대한 성능평가를 진행했으며 모두 적합판정을 받았습니다.
2023. 09. 07Publication
세계 최고 권위 학술지 IEEE 논문 게재 및 특허 취득
Multi-phase computed tomography (CT) is widely adopted for the diagnosis of kidney cancer due to the complementary information among phases. However, the complete set of multi-phase CT is often not available in practical clinical applications. In recent years, there have been some studies to generate the missing modality image from the available data. Nevertheless, the generated images are not guaranteed to be effective for the diagnosis task. In this paper, we propose a unified framework for kidney cancer diagnosis with incomplete multi-phase CT, which simultaneously recovers missing CT images and classifies cancer subtypes using the completed set of images. The advantage of our framework is that it encourages a synthesis model to explicitly learn to generate missing CT phases that are helpful for classifying cancer subtypes. We further incorporate lesion segmentation network into our framework to exploit lesion-level features for effective cancer classification in the whole CT volumes. The proposed framework is based on fully 3D convolutional neural networks to jointly optimize both synthesis and classification of 3D CT volumes. Extensive experiments on both in-house and external datasets demonstrate the effectiveness of our framework for the diagnosis with incomplete data compared with state-of-the-art baselines. In particular, cancer subtype classification using the completed CT data by our method achieves higher performance than the classification using the given incomplete data. <https://pubmed.ncbi.nlm.nih.gov/36327174/>
2022. 12. 08Publication
의료 분야 세계 TOP 저널 네이처 종양학회지 게재
In 2020, it is estimated that 73,750 kidney cancer cases were diagnosed, and 14,830 people died from cancer in the United States. Preoperative multi-phase abdominal computed tomography (CT) is often used for detecting lesions and classifying histologic subtypes of renal tumor to avoid unnecessary biopsy or surgery. However, there exists inter-observer variability due to subtle differences in the imaging features of tumor subtypes, which makes decisions on treatment challenging. While deep learning has been recently applied to the automated diagnosis of renal tumor, classification of a wide range of subtype classes has not been sufficiently studied yet. In this paper, we propose an end-to-end deep learning model for the differential diagnosis of five major histologic subtypes of renal tumors including both benign and malignant tumors on multi-phase CT. Our model is a unified framework to simultaneously identify lesions and classify subtypes for the diagnosis without manual intervention. We trained and tested the model using CT data from 308 patients who underwent nephrectomy for renal tumors. The model achieved an area under the curve (AUC) of 0.889, and outperformed radiologists for most subtypes. We further validated the model on an independent dataset of 184 patients from The Cancer Imaging Archive (TCIA). The AUC for this dataset was 0.855, and the model performed comparably to the radiologists. These results indicate that our model can achieve similar or better diagnostic performance than radiologists in differentiating a wide range of renal tumors on multi-phase CT. <https://www.nature.com/articles/s41698-021-00195-y>
2021. 06. 18연구 및 사업 파트너
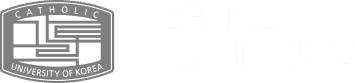

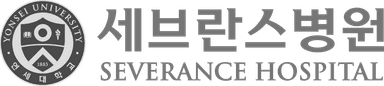
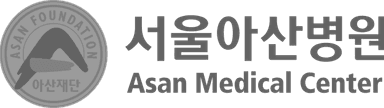

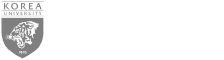
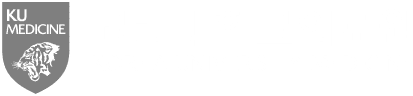
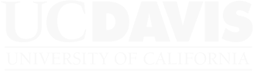

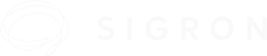
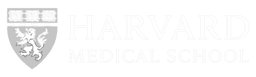